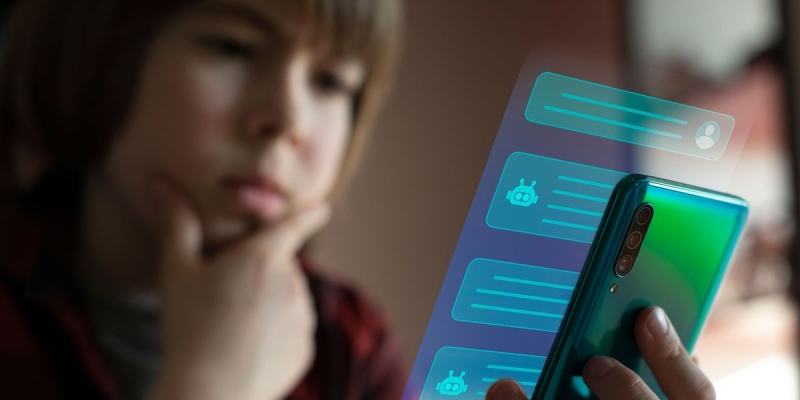
Among the management master Peter Drucker’s a lot of over-quoted turns of expression is “what gets determined gets enhanced.” It’s over-quoted for a factor: It’s real.
No place is it truer than in innovation over the previous 50 years. Moore’s law– which anticipates that the variety of transistors (and for this reason calculate capability) in a chip would double every 24 months– has actually ended up being a self-fulfilling prediction and north star for a whole environment. Since engineers thoroughly determined each generation of making innovation for brand-new chips, they might choose the methods that would approach the objectives of faster and more capable computing. And it worked: Computing power, and more remarkably calculating power per watt or per dollar, has actually grown tremendously in the previous 5 years. The most recent mobile phones are more effective than the fastest supercomputers from the year 2000.
Measurement of efficiency, however, is not restricted to chips. All the parts of our computing systems today are benchmarked– that is, compared to comparable parts in a regulated method, with quantitative rating evaluations. These standards assist drive development.
And we would understand.
As leaders in the field of AI, from both market and academic community, we develop and provide the most extensively utilized efficiency criteria for AI systems on the planet. MLCommons is a consortium that came together in the belief that much better measurement of AI systems will drive enhancement. Because 2018, we’ve established efficiency criteria for systems that have actually revealed more than 50-fold enhancements in the speed of AI training. In 2023, we released our sneak preview standard for big language designs (LLMs), determining the time it required to train a design to a specific quality level; within 5 months we saw repeatable outcomes of LLMs enhancing their efficiency almost threefold. Basically, excellent open standards can move the whole market forward.
We require standards to drive development in AI security
Even as the efficiency of AI systems has actually raced ahead, we’ve seen installing issue about AI security. While AI security indicates various things to various individuals, we specify it as avoiding AI systems from malfunctioning or being misused in damaging methods. AI systems without safeguards might be misused to support criminal activity such as phishing or developing kid sexual abuse product, or might scale up the proliferation of false information or despiteful material. In order to understand the prospective advantages of AI while reducing these damages, we require to drive enhancements in security in tandem with enhancements in abilities.
Our company believe that if AI systems are determined versus typical security goals, those AI systems will get more secure in time. How to robustly and thoroughly assess AI security threats– and likewise track and alleviate them– is an open issue for the AI neighborhood.
Security measurement is challenging since of the numerous various manner ins which AI designs are utilized and the lots of elements that require to be examined. And security is naturally subjective, contextual, and objected to– unlike with unbiased measurement of hardware speed, there is no single metric that all stakeholders settle on for all usage cases.